“Public sentiment is everything. With public sentiment, nothing can fail. Without it, nothing can succeed.” Abraham Lincoln
Mar-tech tools are getting prevalent in enterprises with high-volumes of B2C communication flows. Sentiment Analytics is one such product from the Communications Platform-as-a-Service (CPaaS) suite, which can extract sentiments and provide cues on customer intent from conversational data.
Introduction to Sentiment Analysis
Sentiment analysis, also known as opinion mining, focuses on extracting and analyzing subjective information, emotions, and opinions expressed in text data. Sentiment analysis can be thought of as a detector for understanding people’s feelings and thoughts in written words. It is part of the field of natural language processing (NLP).
Sentiment analysis allows computers to categorize text as positive, negative, or neutral; thereby automating the otherwise time-consuming and error-prone process of manually reviewing and interpreting text. With so many opinions shared online, sentiment analysis helps businesses, marketers and customer success professionals to figure out what people are saying and how they feel.
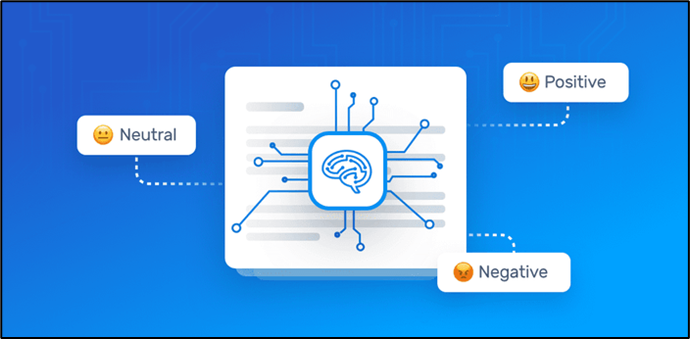
Popular industry applications
a) Social media monitoring
Social media posts often contain some of the most honest opinions about your products, services, and businesses because they are unsolicited.
With the help of sentiment analysis software, you can wade through all that data in minutes, to analyze individual emotions and overall public sentiment on every social platform. Sentiment analysis can read beyond simple definition to detect sarcasm, read common chat acronyms (lol, rofl, etc.), and correct for common mistakes like misused and misspelled words.
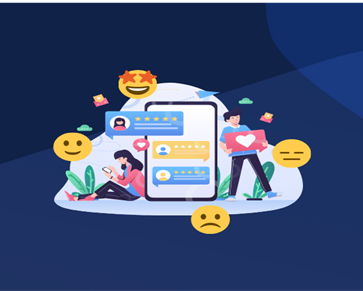
b) Customer support
Customer support management presents many challenges. Sentiment analysis with natural language understanding (NLU) reads regular human language for meaning, emotion, tone, and more, to understand customer requests, just as a person would.
You can automatically process customer support tickets, online chats, phone calls, and emails by sentiment to prioritize any urgent issues. Sentiment analysis could be used to sort thousands of customer support messages instantly by understanding words and phrases that contain negative opinions.
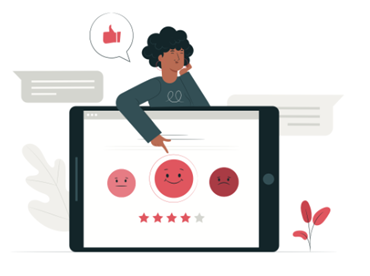
c) Employee satisfaction & Engagement
By analyzing the sentiment of employee feedback, you’ll know how to better engage your employees, reduce turnover, and increase productivity. Use sentiment analysis to evaluate employee surveys or analyze Glassdoor reviews, emails, Slack messages, and more.
By identifying areas of concern or areas where employees are satisfied, organizations can take targeted actions to improve employee engagement, retention, and workplace satisfaction.
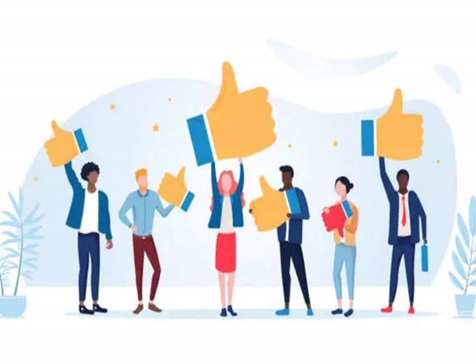
Benefits of using Sentiment Analysis in customer support conversations
- Provide more proactive support: Customers often raise tickets or reach out to customer support when they’re facing a problem. Between the phone calls, emails, and chats you receive, you will have real-time data that allows you to spot recurring issues quickly and fix them before they become unmanageable.
- Avoid biases: The biggest difference between customer support conversations and other data sources is that customers willingly share their feedback and pain points—you don’t need to ask for them, so there is zero possibility of introducing biases in the data.
- Get granular: One of the main advantages of customer support data is that it is all-encompassing. It consists of customer interactions from all customer groups throughout their customer journey—from the time they hear about your product until they make a purchase or leave.

Data privacy concerns
In addressing data privacy concerns in sentiment analysis, several crucial factors must be considered. First and foremost is obtaining informed consent from individuals, ensuring their awareness and agreement regarding data collection and analysis, particularly when dealing with personal information. Anonymization, involving the removal of personally identifiable details, becomes paramount to safeguard privacy, making it impossible to trace sentiments back to specific individuals.
Robust data security measures, such as encryption and secure storage, are imperative to prevent unauthorized access or breaches, upholding the privacy and integrity of sentiment analysis data. Managing bias and ensuring fairness in sentiment analysis models is not only an ethical consideration but also a privacy concern, as biased models may perpetuate stereotypes and discrimination. Lastly, transparency in the sentiment analysis process is crucial, making the methodology understandable to individuals and fostering trust while respecting their privacy.
Connect with customers in a deeper and meaningful way
Sentiment analysis is a powerful force that helps businesses connect with customers on a deeper level. It’s the bridge between data and emotion, driving personalized experiences and informed decision-making. As it continues to evolve, companies must embrace its potential while upholding data privacy. Sentiment analysis isn’t just about text; it’s about understanding and responding to the feelings that shape our world.
About the Author
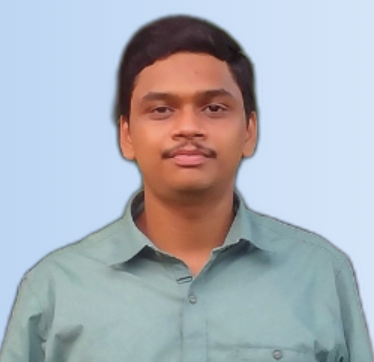
Joel Justin is a computer science engineering student at CMR Institute of Technology in Bangalore. His areas of interest includes programming, database management systems, software testing and digital possibilities.